Myself and my colleague Reju Pillai did a webinar on Anthos and how it can solve industry challenges in the hybrid and multi cloud area. This webinar was done in close collaboration with Google cloud marketing team. We covered the relevance of Anthos and application modernisation to industries in Retail, Digital Natives, Banking and Manufacturing space. Hope that you will find this useful.
GKE Tip series
Kubernetes is the defacto Container orchestration platform today and GKE is a managed Kubernetes distribution from GCP. In addition to being best-in-class Kubernetes distribution, GKE adds all the goodness of GCP to GKE and is also integrated well with the cloud native ecosystem. GKE has been in general availability for the last 5+ years and we have seen customers adopting GKE for many different use cases. Myself and my colleague Reju Pillai decided to do a “GKE Tips series” where our goal is to provide practical tips to GCP practitioners using GKE. Each chapter in the series will cover a specific topic that includes both theoretical aspect and a hands-on demo associated with the topic. We will also provide the steps for you to try this by yourself.
Following are the ones that we have already released. Please feel free to leave feedback on the content as well as topics that you will be interested to see from us.
Right sizing pod memory with GKE Vertical Pod Autoscalar
Secure access to GKE Private clusters
How to choose between GKE Standard, Autopilot and Cloud Run
GKE Usage Metering
Anthos developer sandbox demo
Anthos developer sandbox is pretty cool as it allows developers to experience Kubernetes/GKE/Anthos from right inside their favorite IDE. I made the following demo to showcase how you can use Anthos developer sandbox.
Kubernetes Container Runtimes
I had a recent discussion with my colleagues about Container runtimes supported by Kubernetes, I realized that this topic is pretty complicated with many different technologies and layers of abstractions. As a Kubernetes user, we don’t need to worry about the container runtime used below Kubernetes for most practical purposes but as an engineer, its always good to know what’s happening under the hood.
Following picture shows the relationship between how Kubernetes and Containers are tied together through Container Runtime Interface(CRI)
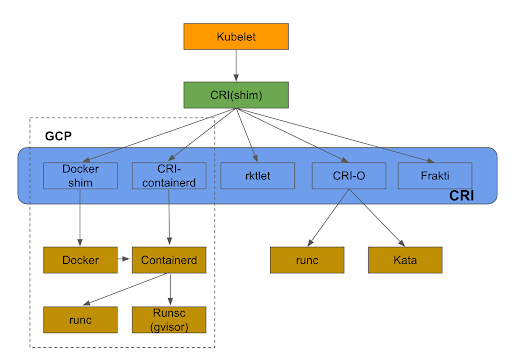
Following are some notes around this:
- Container runtime is a software that implements and manages containers.
- OCI(Open container initiative) has released a standard for building container images and for running containers. runc is an implementation of OCI container runtime specification.
- CRI layer allows Kubernetes to talk to any Container runtime including Docker, Rkt.
- GKE supports Docker, Containerd as Container runtimes. Docker is an abstraction on top of Containerd.
- GVisor project allows for running secure containers by providing and additional layer of kernel abstraction.
- CRI-O is an CNCF project that leverages OCI standards for runtime, images and networking.
Kubernetes and GKE learning series
I recently did a 4 week Kubernetes and GKE learning series in Google cloud. The goal here was to take some of the fundamental Kubernetes and GKE concepts and run them through with specific Qwiklabs to illustrate the concepts. Qwiklabs is a GCP sandbox environment to try out GCP technologies. The nice thing with Qwiklabs is that there is no need to even signup for a GCP account. Another good thing with Qwiklabs is that the same instructions can be tried out in your personal GCP account as well.
Following are the recordings to the series:
Migrating an application from Virtual machine to Containers and run it in GKE
Securing Access in Kubernetes/GKE
Troubleshooting with GKE
Running Serverless applications on GKE
Please provide your feedback and also let me know if there are any further topics that you will be interested.
Tech Agility conference
I recently delivered a session on “Best practises for using Kubernetes in production” in Technical Agility conference. Considering that it’s COVID time, the conference itself was virtual. The conference was well organised and I enjoyed the Q&A session. Following is an abstract of my topic:
Kubernetes has become the de facto Container orchestration platform over the last few years. For all the power and the scale that Kubernetes provides, it’s still a complex platform to configure and manage. Managed Kubernetes service like GKE takes a lot of the pain out in managing Kubernetes. When we are using Kubernetes in production, it makes sense to follow the best practises around distributed system development targeted towards Kubernetes. In this session, I will talk about Kubernetes and GKE best practices around infrastructure, security and applications with specific focus on day-2 operations.
Following is the link to the presentation:
Recording:
Techgig Kubernetes Webinar
Recently, I did a webinar with Techgig on “Kubernetes design principles, patterns and ecosystem”. In this webinar, I covered the following topics:
- Key design principles behind Kubernetes
- Common design patterns with pods
- Day-2 Kubernetes best practises
- Kubernetes ecosystem
Following link has the recording to the webinar. I have also added a link to the slides below.
Kubernetes and GKE – Day 2 operations
For many folks working with Containers and Kubernetes, the journey begins with trying few sample container applications and then deploying applications into production in a managed kubernetes service like GKE. GKE or any managed kubernetes services provides lot of features and controls and it is upto the user to leverage them the right way. Based on my experience, what I see is that the best practises are not typically followed which results in post-production issues. There are some parameters that cannot be changed post cluster creation and this makes the problem even more difficult to handle. In this blog, I will share a set of resources that cover the best practises around Kubernetes and GKE. If these are evaluated before cluster creation and a proper design is done before-hand, it will prevent a lot of post-production issues.
This link talks about best practises with building containers and this link talks about best practises with operating containers. This will create a strong foundation around Containers.
Following collection of links talk about the following best practises with Kubernetes. These are useful both from developer and operator perspective.
- Building small container images
- Organizing with namespaces
- Using healthchecks
- Setting up resource limits for containers
- Handling termination requests gracefully
- Talking to external services outside Kubernetes
- Upgrading clusters with zero downtime
Once we understand best practises around Docker and Kubernetes, we need to understand the best practises around GKE. Following set of links cover these well:
- Preparing GKE environment for production
- Guidelines for scalable clusters
- GKE security best practises
- Best practises to optimize cost with GKE
- CIS Kubernetes and GKE benchmark
If you are looking for GKE samples to try, this is a good collection of GKE samples. These are useful to play around kubernetes without writing a bunch of yaml..
GCP provides qwiklabs to try out a particular GCP concept/feature in a sandboxed environment. Following qwiklabs quests around Kubernetes and GKE are very useful to get hands-on experience. Each quest below has a set of labs associated with that topic.
- Kubernetes in Google cloud
- Deploy to kubernetes in Google cloud
- GKE best practices
- GKE best practises – Security
- Kubernetes solutions
For folks looking for free Kubernetes books to learn, I found the following 3 books to be extremely useful. The best part about them is they are free to download.
Following is a good course in Coursera for getting started in GKE.
Please feel free to ping me if you find other useful resources around getting your containers to production.
Kubernetes DR
Recently, we had a customer issue where a production GKE cluster was deleted accidentally which caused some outage till the cluster recovery was completed. Recovering the cluster was not straightforward as the customer did not have any automated backup/restore mechanism and also the presence of stateful workloads complicated this further. I started looking at some of the ways in which a cluster can be restored to a previous state and this blog is a result of that work.
Following are some of the reasons why we need DR for Kubernetes cluster:
- Cluster is deleted accidentally.
- Cluster master node has gotten into a weird state. Having redundant masters would avoid this problem.
- Need to move from 1 cluster type to another. For example, GKE legacy network to VPC native network migration.
- Move to different kubernetes distribution. This can include moving from onprem to cloud.
The focus of this blog is more from a cold DR perspective and to not have multiple clusters working together to provide high availability. I will talk about multiple clusters and hot DR in a later blog.
There are 4 kinds of data to backup in a kubernetes cluster:
- Cluster configuration. These are parameters like node configuration, networking and security constructs for the cluster etc.
- Common kubernetes configurations. Examples are namespaces, rbac policies, pod security policies, quotas, etc.
- Application manifests. This is based on the specific application that is getting deployed to the cluster.
- Stateful configurations. These are persistent volumes that is attached to pods.
For item 1, we can use any infrastructure automation tools like Terraform or in the case of GCP, we can use deployment manager. The focus of this blog is on items 2, 3 and 4.
Following are some of the options possible for 2, 3 and 4:
- Use a Kubernetes backup tool like Velero. This takes care of backing up both kubernetes resources as well as persistent volumes. This covers items 2, 3 and 4, so its pretty complete from a feature perspective. Velero is covered in detail in this blog.
- Use GCP “Config sync” feature. This can cover 2 and 3. This approach is more native with Kubernetes declarative approach and the config sync approach tries to recreate the cluster state from stored manifest files. Config sync approach is covered in detail in this blog.
- Use CI/CD pipeline. This can cover 2 and 3. The CI/CD pipeline typically does whole bunch of other stuff in the pipeline and it is a roundabout approach to do DR. An alternative could be to create a separate DR pipeline in CI/CD.
- Kubernetes volume snapshot and restore feature was introduced in beta in 1.17 release. This is targeted towards item 4. This will get integrated into kubernetes distributions soon. This approach will use kubernetes api itself to do the volume snapshot and restore.
- Manual approach can be taken to backup and restore snapshots as described here. This is targeted towards item 4. The example described here for GCP talks about using cloud provider tool to take a volume snapshot , create a disk from the volume and then manually create a PV and attach the disk to the PV. The kubernetes deployment can use the new PV.
- Use backup and restore tool like Stash. This is targeted towards item 4. Stash is a pretty comprehensive tool to backup Kubernetes stateful resources. Stash provides a kubernetes operator on top of restic. Stash provides add-ons to backup common kubernetes stateful databases like postgres, mysql, mongo etc.
I will focus on Velero and Config sync in this blog.
Following is the structure of the content below. The examples are tried on GKE cluster.
Velero
Velero was previously Heptio Ark. Velero provides following functionalities:
- Manual as well as periodic backups can be scheduled. Velero can backup and restore both kubernetes resources as well as persistent volumes.
- Integrated natively with Amazon EBS Volumes, Azure Managed Disks, Google Persistent Disks using plugins. For some storage systems like Portworx, there is a community supported provider. Velero also integrates with Restic open source project that allows integration with any provider. This link provides complete list of supported providers.
- Can handle snapshot consistency problem by providing pre and post hooks to flush the data before snapshot is taken.
- Backups can be done for the complete cluster or part of the cluster like at individual namespace level.
Velero follows a client, server model. The server needs to be installed in the GKE cluster. The client can be installed as a standalone binary. Following are the installation steps for the server:
- Create Storage bucket
- Create Service account. The storage account needs to have enough permissions to create snapshots and also needs to have access to storage bucket
- Install velero server
Velero Installation
For the client component, I installed it in mac using brew.
brew install velero
For the server component, I followed the steps here.
Create GKE cluster
gcloud beta container --project "sreemakam-test" clusters create "prodcluster" --zone "us-central1-c" --enable-ip-alias
Create storage bucket
BUCKET=sreemakam-test-velero-backup
gsutil mb gs://$BUCKET/
Create service account with right permissions
gcloud iam service-accounts create velero \ --display-name "Velero service account"
SERVICE_ACCOUNT_EMAIL=$(gcloud iam service-accounts list \ --filter="displayName:Velero service account" \ --format 'value(email)')
ROLE_PERMISSIONS=( compute.disks.get compute.disks.create compute.disks.createSnapshot compute.snapshots.get compute.snapshots.create compute.snapshots.useReadOnly compute.snapshots.delete compute.zones.get ) gcloud iam roles create velero.server \ --project $PROJECT_ID \ --title "Velero Server" \ --permissions "$(IFS=","; echo "${ROLE_PERMISSIONS[*]}")" gcloud projects add-iam-policy-binding $PROJECT_ID \ --member serviceAccount:$SERVICE_ACCOUNT_EMAIL \ --role projects/$PROJECT_ID/roles/velero.server
Download service account locally
This service account is needed for the installation of Velero server.
gcloud iam service-accounts keys create credentials-velero \ --iam-account $SERVICE_ACCOUNT_EMAIL
Set appropriate bucket permission
gsutil iam ch serviceAccount:$SERVICE_ACCOUNT_EMAIL:objectAdmin gs://${BUCKET}
Install Velero server
This has to be done after setting the right Kubernetes context.
velero install \
--provider gcp \
--plugins velero/velero-plugin-for-gcp:v1.0.1 \
--bucket $BUCKET \
--secret-file ./credentials-velero
After this, we can check that Velero is successfully installed:
$ velero version
Client:
Version: v1.3.1
Git commit: -
Server:
Version: v1.3.1
Install application
To test the backup and restore feature, I have installed 2 Kubernetes application, the first is a hello go based stateless application and the second is stateful wordpress application. I have forked the GKE examples repository and made some changes for this use case.
Install hello application
kubectl create ns myapp
kubetcl apply -f hello-app/manifests -n myapp
Install wordpress application
First, we need to create sql secrets and then we can apply k8s manifests
SQL_PASSWORD=$(openssl rand -base64 18)
kubectl create secret generic mysql -n myapp \
--from-literal password=$SQL_PASSWORD
kubectl apply -f wordpress-persistent-disks -n myapp
This application has 2 stateful resource, 1 for mysql persistent disk and another for wordpress. To validate the backup, open the wordpress page, complete the basic installation and create a test blog. This can be validated as part of restore.
Resources created
$ kubectl get secrets -n myapp
NAME TYPE DATA AGE
default-token-cghvt kubernetes.io/service-account-token 3 22h
mysql Opaque 1 22h
$ kubectl get deployments -n myapp
NAME READY UP-TO-DATE AVAILABLE AGE
helloweb 1/1 1 1 22h
mysql 1/1 1 1 21h
wordpress 1/1 1 1 21h
$ kubectl get services -n myapp
NAME TYPE CLUSTER-IP EXTERNAL-IP PORT(S) AGE
helloweb LoadBalancer 10.44.6.6 34.68.231.47 80:31198/TCP 22h
helloweb-backend NodePort 10.44.4.178 <none> 8080:31221/TCP 22h
mysql ClusterIP 10.44.15.55 <none> 3306/TCP 21h
wordpress LoadBalancer 10.44.2.154 35.232.197.168 80:31095/TCP 21h
$ kubectl get ingress -n myapp
NAME HOSTS ADDRESS PORTS AGE
helloweb * 34.96.67.172 80 22h
$ kubectl get pvc -n myapp
NAME STATUS VOLUME CAPACITY ACCESS MODES STORAGECLASS AGE
mysql-volumeclaim Bound pvc-3ebf86a0-8162-11ea-9370-42010a800047 200Gi RWO standard 21h
wordpress-volumeclaim Bound pvc-4017a2ab-8162-11ea-9370-42010a800047 200Gi RWO standard 21h
$ kubectl get pv -n myapp
kNAME CAPACITY ACCESS MODES RECLAIM POLICY STATUS CLAIM STORAGECLASS REASON AGE
pvc-3ebf86a0-8162-11ea-9370-42010a800047 200Gi RWO Delete Bound myapp/mysql-volumeclaim standard 21h
pvc-4017a2ab-8162-11ea-9370-42010a800047 200Gi RWO Delete Bound myapp/wordpress-volumeclaim standard
Backup kubernetes cluster
The backup can be done at the complete cluster level or for individual namespaces. I will create a namespace backup now.
$ velero backup create myapp-ns-backup --include-namespaces myapp
Backup request "myapp-ns-backup" submitted successfully.
Run `velero backup describe myapp-ns-backup` or `velero backup logs myapp-ns-backup` for more details.
We can look at different commands like “velero backup describe”, “velero backup logs”, “velero get backup” to get the status of the backup. The following output shows that the backup is completed.
$ velero get backup
NAME STATUS CREATED EXPIRES STORAGE LOCATION SELECTOR
myapp-ns-backup Completed 2020-04-19 14:35:51 +0530 IST 29d default <none>
Let’s look at the snapshots created in GCP.
$ gcloud compute snapshots list
NAME DISK_SIZE_GB SRC_DISK STATUS
gke-prodcluster-96c83f-pvc-7a72c7dc-74ff-4301-b64d-0551b7d98db3 200 us-central1-c/disks/gke-prodcluster-96c83f-pvc-3ebf86a0-8162-11ea-9370-42010a800047 READY
gke-prodcluster-96c83f-pvc-c9c93573-666b-44d8-98d9-129ecc9ace50 200 us-central1-c/disks/gke-prodcluster-96c83f-pvc-4017a2ab-8162-11ea-9370-42010a800047 READY
Let’s look at the contents of velero storage bucket:
gsutil ls gs://sreemakam-test-velero-backup/backups/
gs://sreemakam-test-velero-backup/backups/myapp-ns-backup/
When creating snapshots, it is necessary that the snapshots are created in a consistent state when the writes are in the fly. The way Velero achieves this is by using backup hooks and sidecar container. The backup hook freezes the filesystem when backup is running and then unfreezes the filesystem after backup is completed.
Restore Kubernetes cluster
For this example, we will create a new cluster and restore the contents of namespace “myapp” to this cluster. We expect that both the kubernetes manifests as well as persistent volumes are restored.
Create new cluster
$ gcloud beta container --project "sreemakam-test” clusters create "prodcluster-backup" --zone "us-central1-c" --enable-ip-alias
Install Velero
velero install \
--provider gcp \
--plugins velero/velero-plugin-for-gcp:v1.0.1 \
--bucket $BUCKET \
--secret-file ./credentials-velero \
--restore-only
I noticed a bug that even though we have done the installation with “restore-only” flag, the storage bucket is mounted as read-write. Ideally, it should be only “read-only” so that both clusters don’t write to the same backup location.
$ velero backup-location get
NAME PROVIDER BUCKET/PREFIX ACCESS MODE
default gcp sreemakam-test-velero-backup ReadWrite
Let’s look at the backups available in this bucket:
$ velero get backup
NAME STATUS CREATED EXPIRES STORAGE LOCATION SELECTOR
myapp-ns-backup Completed 2020-04-19 14:35:51 +0530 IST 29d default <none>
Now, let’s restore this backup in the current cluster. This cluster is new and does not have any kubernetes manifests or PVs.
$ velero restore create --from-backup myapp-ns-backup
Restore request "myapp-ns-backup-20200419151242" submitted successfully.
Run `velero restore describe myapp-ns-backup-20200419151242` or `velero restore logs myapp-ns-backup-20200419151242` for more details.
Let’s make sure that the restore is completed successfully:
$ velero restore get
NAME BACKUP STATUS WARNINGS ERRORS CREATED SELECTOR
myapp-ns-backup-20200419151242 myapp-ns-backup Completed 1 0 2020-04-19 15:12:44 +0530 IST <none>
The restore command above would create all the manifests including namespaces, deployments and services. It will also create PVs and attach to the appropriate pods.
Let’s look at the some of the resources created:
$ kubectl get services -n myapp
NAME TYPE CLUSTER-IP EXTERNAL-IP PORT(S) AGE
helloweb LoadBalancer 10.95.13.226 162.222.177.146 80:30693/TCP 95s
helloweb-backend NodePort 10.95.13.175 <none> 8080:31555/TCP 95s
mysql ClusterIP 10.95.13.129 <none> 3306/TCP 95s
wordpress LoadBalancer 10.95.7.154 34.70.240.159 80:30127/TCP 95s
$ kubectl get pv -n myapp
NAME CAPACITY ACCESS MODES RECLAIM POLICY STATUS CLAIM STORAGECLASS REASON AGE
pvc-3ebf86a0-8162-11ea-9370-42010a800047 200Gi RWO Delete Bound myapp/mysql-volumeclaim standard 5m18s
pvc-4017a2ab-8162-11ea-9370-42010a800047 200Gi RWO Delete Bound myapp/wordpress-volumeclaim standard 5m17s
We can access the “hello” application service and “wordpress” and it should work fine. We can also check that the test blog created earlier is restored fine.
Config sync
GCP “Config sync” feature provides gitops functionality to kubernetes manifests. “Config sync” feature is installed as Kubernetes operator. When Config sync operator is installed in Kubernetes cluster, it points to a repository that holds the kubernetes manifests. The config sync operator makes sure that the state of the cluster reflects what is mentioned in the repository. Any changes to the local cluster or to the repository will trigger reconfiguration in the cluster to sync from the repository. “Config sync” feature is a subset of Anthos config management(ACM) feature in Anthos and it can be used without Anthos license. ACM provides uniform configuration and security policies across multiple kubernetes clusters. In addition to providing config sync functionality, ACM also includes policy controller piece that is based on opensource gatekeeper project.
Config sync feature can be used for 2 purposes:
- Maintain security policies of a k8s cluster. The application manifests can be maintained through a CI/CD system.
- Maintain all k8s manifests including security policies and application manifests. This approach allows us to restore cluster configuration in a DR scenario. The application manifests can still be maintained through a CI/CD system, but using CI/CD for DR might be time consuming.
In this example, we will use “Config sync” for DR purposes. Following are the components of “Config sync” feature:
- “nomos” CLI to manage configuration sync. It is possible that this can be integrated with kubectl later.
- “Config sync” operator installed in the kubernetes cluster.
Following are the features that “Config sync” provides:
- Config sync works with GCP CSR(code source repository), bitbucket, github, gitlab
- With namespace inheritance, common features can be put in abstract namespace that applies to multiple namespaces. This is useful if we want to share some kubernetes manifests across multiple clusters.
- Configs for specific clusters can be specified using cluster selector
- Default sync period is 15 seconds and it can be changed.
The repository follows the structure as below. The example below shows a sample repo with the following folders(cluster->cluster resources like quotas, rbac, security policy etc, clusterregistry->policies specific to each cluster, namespaces->application manifest under each namespace, system->operator related configs)

Following are the steps that we will do below:
- Install “nomos” CLI
- Checkin configs to a repository. We will use github for this example.
- Create GKE cluster, make current user cluster admin. To access private git repo, we can setup kubernetes secrets. For this example, we will use public repository.
- Install config management CRD in the cluster
- Check nomos status using “nomos status” to validate that the cluster has synced to the repository.
- Apply kubernetes configuration changes to the repo as well as to cluster and check that the sync feature is working. This step is optional.
Installation
I installed nomos using the steps mentioned here in my mac.
Following commands download and install the operator in the GKE cluster. I have used the same cluster created in the “Velero” example.
gsutil cp gs://config-management-release/released/latest/config-sync-operator.yaml config-sync-operator.yaml
kubectl apply -f config-sync-operator.yaml
To verify that “Config sync” is running correctly, please check the following output. We should see that the pod is running successfully.
$ kubectl -n kube-system get pods | grep config-management
config-management-operator-5d4864869d-mfrd6 1/1 Running 0 60s
Let’s check the nomos status now. As we can see below, we have not setup the repo sync yet.
$ nomos status --contexts gke_sreemakam-test_us-central1-c_prodcluster
Connecting to clusters...
Failed to retrieve syncBranch for "gke_sreemakam-test_us-central1-c_prodcluster": configmanagements.configmanagement.gke.io "config-management" not found
Failed to retrieve repos for "gke_sreemakam-test_us-central1-c_prodcluster": the server could not find the requested resource (get repos.configmanagement.gke.io)
Current Context Status Last Synced Token Sync Branch
------- ------- ------ ----------------- -----------
* gke_sreemakam-test_us-central1-c_prodcluster NOT CONFIGURED
Config Management Errors:
gke_sreemakam-test_us-central1-c_prodcluster ConfigManagement resource is missing
Repository
I have used the repository here and plan to syncup the “gohellorepo” folder. Following is the structure of the “gohellorepo” folder.
$ tree .
.
├── namespaces
│ └── go-hello
│ ├── helloweb-deployment.yaml
│ ├── helloweb-ingress.yaml
│ ├── helloweb-service.yaml
│ └── namespace.yaml
└── system
└── repo.yaml
The repository describes a namespace “go-hello” and the “go-hello” directory contains kubernetes manifests for a go application.
The repository also has the “config-management.yaml” file that describes the repo that we want to sync to. Following is the content fo the file:
# config-management.yaml
apiVersion: configmanagement.gke.io/v1
kind: ConfigManagement
metadata:
name: config-management
spec:
# clusterName is required and must be unique among all managed clusters
clusterName: my-cluster
git:
syncRepo: https://github.com/smakam/csp-config-management.git
syncBranch: 1.0.0
secretType: none
policyDir: "gohellorepo"
As we can see, we want to sync to the “gohellorepo” folder in git repo “https://github.com/smakam/csp-config-management.git”
Syncing to the repo
Following command syncs the cluster to the github repository:
kubectl apply -f config-management.yaml
Now, we can look at “nomos status” to check if the sync is successful. As we can see from “SYNCED” status, the sync is successful.
$ nomos status --contexts gke_sreemakam-test_us-central1-c_prodcluster
Connecting to clusters...
Current Context Status Last Synced Token Sync Branch
------- ------- ------ ----------------- -----------
* gke_sreemakam-test_us-central1-c_prodcluster SYNCED 020ab642 1.0.0
Let’s look at the kubernetes resources to make sure that the sync is successful. As we can see below, the namespace and the appropriate resources got created in the namespace “go-hello”
$ kubectl get ns
NAME STATUS AGE
config-management-system Active 23m
default Active 27h
go-hello Active 6m26s
$ kubectl get services -n go-hello
NAME TYPE CLUSTER-IP EXTERNAL-IP PORT(S) AGE
helloweb LoadBalancer 10.44.6.41 34.69.102.8 80:32509/TCP 3m25s
helloweb-backend NodePort 10.44.13.224 <none> 8080:31731/TCP 3m25s
$ kubectl get deployments -n go-hello
NAME READY UP-TO-DATE AVAILABLE AGE
helloweb 1/1 1 1 7m
As a next step, we can make changes to the repo and check that the changes are pushed to the cluster. If we make any manual changes. to the cluster, config sync operator will check with the repo and push the changes back to the cluster. For example, if we delete namespace “go-hello” manually in the cluster, we will see that after 30 seconds or so, the namespace configuration is pushed back and recreated in the cluster.
References
Service to service communication within GKE cluster
In my last blog, I covered options to access GKE services from external world. In this blog, I will cover service to service communication options within GKE cluster. Specifically, I will cover the following options:
- Cluster IP
- Internal load balancer(ILB)
- Http internal load balancer
- Istio
- Traffic director
In the end, I will also compare these options and suggest matching requirement to a specific option. For each of the options, I will deploy a helloworld service with 2 versions and then have a client access the hello service. The code that includes manifest files for all the options is available in my github project here.